Article Sections –
- Introduction
- Inspiration
- The Idea of Personalization
- Evolution of user experience with repeated personalization
- Monetary benefits of personalization
- Benefit to current recommendation applications
- Patenting the approach
- Noteworthy current changes
Introduction – Food and its associated services constitute one of the world’s largest industries approximated at 10% of global GDP, around $8 trillion. In this, the food recommendation apps play an integral role in connecting food to its consumers. Apps such as Foursquare, OpenTable, Zomato, Yelp, Swiggy, Google reviews and Grubhub help us decide where to eat next or what to try at a new restaurant.
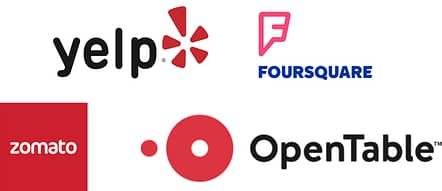
Image 1: Some common restaurant recommendation applications.
Note: Images only represent common food recommendation apps. No copyright infringement is intended.
Almost everyone with a smartphone has utilized at least one of these apps, allowing them to inform us which nearby restaurant to go to or which dish has the highest rating. A majority of these apps allow users to post their experiences of the food, the ambience and the service at the restaurant they visit. These reviews build a database that can be tapped into when a new customer seeks similar information. Ideally, the more positive reviews a restaurant and its food receives, the higher up the recommendation list it advances. This allows more app-users to discover the restaurant and leads to an increase in foot traffic.
To get personal for a moment, I am a vegetarian and am always on the lookout for new kinds of vegetarian tastes and cuisines around. Unsurprisingly, I have used a number of restaurant and food recommendation apps to make my decisions. However, almost all of them leave me with a feeling of incomplete assistance. After being unable to ignore my dissatisfaction, I decided to pen down attributes that a comprehensive food recommendation app could benefit from.
With the current restaurant recommendation apps, I am forced to rate restaurants publicly just to keep a record of restaurants I have been to. While public ratings and reviews help get clicks and eyeballs for the app, the restaurant and the review, the user completely misses the experience of storing private information about the event. Overall, I believe that a comprehensive food recommendation app is yet to come. This comprehensive food recommendation app must go beyond the public profile and give us a chance to personalize our experience.
Towards this, I propose a new kind of app that coalesces what I consider three critical aspects of the “eating out” experience and beyond. They are:
- Personalization
- Food Tracking
- Meal Kit Service
Inspiration – The inspiration to think about this problem came from one of my visits to a Chinese cuisine restaurant in Phoenix. While I deeply enjoyed almost all of their vegetarian menu items, I did not like two of the entrees. I decided that I should remember the menu items that I did not like and not repeat the order on my next visit. However, upon my visit after 6 months, I absolutely failed to remember what I previously disliked and ended up ordering the same items. Disappointed by the situation, I then checked a number of restaurant recommendation apps and failed to find any section that allows personalizing an experience. Hence, this blog article.
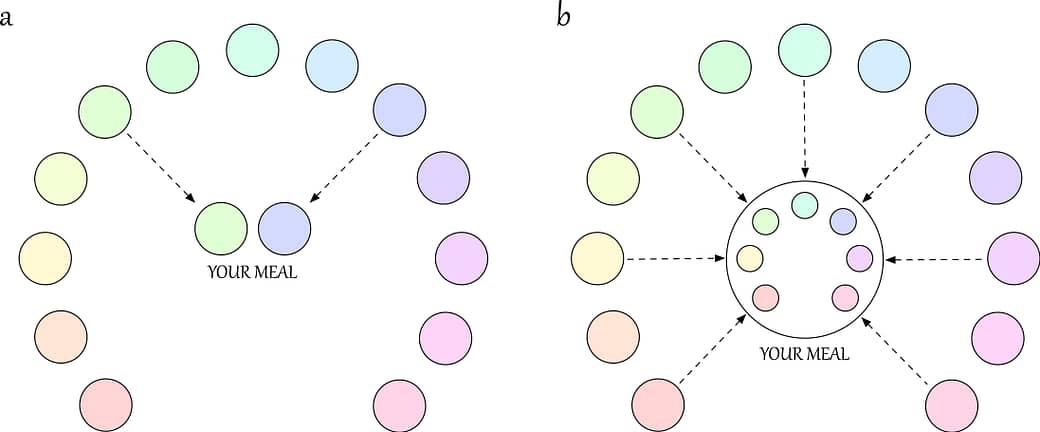
Image 2: Categories of food experience at any restaurant (a) Order one or more menu items (Examples: IHOP, California Pizza Kitchen) and (b) build your meal (Examples: Souplantation, Genghis Grill, Chipotle)
A meal at any restaurant can be broadly classified into two categories. Either the customer orders one or more of the menu items from the list (Examples: IHOP, California pizza kitchen) (Image 2a) or builds his/her meal in a buffet or make your own salad/grill (Examples: Souplantation / Genghis grill / Chipotle) (Image 2b). It is important to note these two categories, as we shall revisit them later in the article and explain how personalization could apply to both these categories.
Challenge: To reiterate, there is no convenient way to take private notes about your experience at a restaurant to remember your likes and dislikes at the place. It is time to change that!
The Idea of Personalization – Eating out is one of the more personal experiences with friends and family. It is almost certainly a cornerstone decision to celebrate any happy occasion. Be it an inconsequential decision or an eventful one, increased personalization in restaurant recommendation apps could help us all.
We need a system to be able to take easy private notes about our experiences at restaurants, our likes, dislikes, and idiosyncrasies. For example, a personal choice of spice level preferred in a dish doesn’t need to be broadcasted via a public profile. However, this information needs to be stored so it can be referenced for future visits. A comprehensive food recommendation app could address these challenges and benefit users by considering the following information:
- A table with a great view. This information could be saved in an app using the physical location or a number
- Whether a specific dish suites our gastrointestinal physiology
- Personal choices communicated at the restaurant (spice level, special side dish requests, music with the food, chef’s recommendations etc)
- Allow reminders to be set to visit a restaurant to grab a special seasonal dish.
- Record the combinations of ingredients, spices and sauces used in restaurants like Genghis Grill (A build your own stir fry place) or Souplantation (build your own salad) that suit your palate.
Taking a fictional food recommendation app as an example, I would like to provide a design system of how the personalization of a restaurant app could work.
Category 1 – Order food from a list of items
Step 1. (First-time users) Toggling between Public and Personal Profiles
Upon opening the application on the phone, the user is transported to his/her public profile. This is the profile that opens by default once a user opens the app. Next, the user conducts the search until he/she finds the restaurant of choice and the menu items based on existing public reviews and recommendations (#1-3). Once the user orders and enjoys the items of choice, it is time to take note of the personal experience. At this point, the user can swipe down to toggle between the public and personal profiles as shown below (#3).
Step 2 – Personalizing the experience (Accessing the menu)
Once the user switches to the personal profile, the app confirms the specified restaurant to initiate accessing the menu options. The menu information can be obtained by standard web crawling applications or uploaded by the restaurant owner.
Upon confirming those questions the user is taken to the menu where itemized listings can be accessed (starting from appetizers, ending at the desserts etc). The selection of a menu item takes the user deeper within each item (#4-6).
Step 3 – Personalizing the experience (Note taking)
Selecting the specified menu item from the list takes the user deeper into the selection to begin the note-taking. The user is confronted with a series of questions that capture the essence of the experience. Icons such as a camera could help the user keep a pictographic memory of the menu item/event if desired (#7-9). The series of questions ends into a space to take custom notes about the experience. This is the place where the user could note additional information that the questions were unable to capture.
These questions could be customizable to generate a personalized experience. The user could select the questions from a list that seems important to him/her to judge a restaurant experience.
Foods have long been known to trigger health conditions such as inflammatory bowel disease, irritable bowel syndrome, food poisoning, migraine and colitis (1-4). Apps such as GI buddy, Cara app, mySymptoms Food Diary & Symptom Tracker help users keep track of the foods that trigger incidences of their chronic conditions. However, these apps do not allow easy universal tracking experience such as the one proposed here.
The user could choose to add relevant questions that ask specific questions about their chronic conditions as shown below. In addition, the app could give users the ability to design their own questions that can be answered by Y/N/Maybe (via fill in the blanks). As it is impossible to accurately determine the desires of every individual, giving a user the ability to design their own questions with strict categorical replies could rapidly improve the relevance and usability of the mobile application while maintaining the ease in data mining. These questions could further reflect the user’s need to track their personalized questions. These questions could be added to any menu item selection by a long press on the screen that brings up the pool of fill in the blanks.
Other relevant questions that could aid in food tracking answered by Yes/No/Maybe –
- Was the food low sodium / low potassium?
- Was the restaurant kid friendly?
- Was the meal too expensive?
The answers to these highly personalized categorical questions could be used to create a useful personalized private database. This data could then be locally mined to understand user specific habits, likes and dislikes and provide personalized food and restaurant recommendations.
The user could also be provided with information regarding potential foods that might trigger their disease condition. The information could be mined to identify specific ingredients within the menu items that could trigger the disease/allergic response. The output data could help generate a personalized list of ingredients, ingredient combinations and foods that trigger diseases to be communicated to the user. Further, the singular user data could be anonymously combined with multiple users to create a global database of triggers for specific conditions.
Step 4 – Accessing an already written review (Repeat user)
As the user scrolls down the menu, he/she might encounter a previously ordered and reviewed menu item such as the one shown in #10.
The app could give important relevant information such as the number of reviews and average score right next to the menu item. Tapping on the number of review complication could open the dates on which the menu item was ordered and reviewed by the user (as shown in #11).
Selecting any specific date brings the user deeper into the menu item and shows them their answers to the specific questions they previously answered (#12-13). Importantly, the user is also shown the notes that he/she might have taken while reviewing the menu item during the previous visit. These notes could help the customer to decide whether to order the menu item again or to go with another option. These notes could also help the user understand how their own tastes and the specific menu item has changed over time.
Once the user completes adding all the components to his/her meal, the app allows the user to simply click next until the summary page is reached to complete the process. The user could then exit the personal page by toggling back to the public profile page (#14-16).
Category 2 – Build your meal (Salad bar / Grill)
The second category of restaurants allows customers to build their own meal by from a selection of ingredients that the customers put together in their bowl or burrito (Examples: Experiences at Chipotle, Souplantation, Genghis Grill etc). These menu items are more customizable and offer a challenge on how to be tracked.
The app could provide information about all the ingredients to the user in a logical sequence. The user could simply add all the ingredients that he/she has used to build the meal and rate the experience thereafter. The personalized interface of the app could open to allow the user to visit their previous recorded experiences. In addition, the welcome page of the app could provide information on how the user has rated the restaurant on the previous visits (taste analytics) and suggest recommendations based on that for the current visit.
As the user moves on to make the bowl and rate the experience, the user could be presented with the list of individual items that are a part of the meal. As a part of the personalization, the user could begin to tap on the ingredients to add them to the meal. As the user taps on a specific ingredient, it is registered as a part of the meal. The user could simply tap the menu item multiple times to indicate a higher quantity of the component added. To undo the number, the user could simply press back and change the number back.Taking the example of a fictional make your own Grill restaurant I suggest the following approach to the entire experience. The user adds items selected across different sections of the menu.
Once the user completes the addition, the Next button takes the user to the summary section of the items and proceeds to the place to take notes on the experience (#6).
Once the user completes summarizing the meal, the next selection takes the user to the place to rate the experience of the meal with an option of taking special notes. More questions can be added as before. The summary experience is now added to the list of all the previous visits to the restaurant.
The user could also access taste analytics to see the updated history of the ratings and delve deep into what could be the most liked or disliked items from the offering (recommendation engine). An algorithm to track the changes in the menu items across different visits could help estimate the likes and dislikes. Known interactions between different additions in the meal could help improve the accuracy of the recommendation engine.
How these interactions could evolve over time and enrich the user experience?
With time and repeated recording of likes and dislikes the algorithms can continue to learn the user behavior to produce improved, personalized and accurate recommendations. The user could then begin to seek recommendations about individual menu items, restaurants based on these enriched understandings. Based on the history of a user at a restaurant, the user could see his/her highest-rated menu items/selections to avoid or enjoy.
Public pooling of these datasets could also allow highly customizable user searches as well. Currently, the search choices available to any user are limited to a small number such as vegetarian, vegan, gluten-free, clean fish, lean meats etc. The aggregated rich public database could be mined to help the user search for complex combinations of keywords such as restaurants and menu items that are rated IBD trigger free and migraine free, low sodium/potassium meals in high ambience restaurant etc.
How can this addition bring monetary benefit to the app?
1. Data as a service to restaurant owners/market analysts (with security and user privacy): User data could be securely trimmed down and provided to the restaurant owners/market analysts for a fee. As the user highly personalizes the experience with categorical responses, they can be grouped according to the menu item/restaurant chain in the data package that is sold to the owner/market analyst. The user details need not come into the picture at any point, hence maintaining the user privacy.
2. User condition tracking: Social media already plays a major role in quickly identifying restaurants that caused an outbreak of foodborne illnesses such as norovirus infection at Chipotle. Customized tracking could also allow new food items and restaurants that trigger episodes of chronic conditions such as IBD, colitis and migraine.
3. Meal kit options: The app could also team up with meal kit vendors such as Blue Apron, Plated and Amazon Fresh to suggest personalized choices that the user might like based on the user ratings, recommendation engine suggestions after the meal. An example is shown below.
4. Personalization based on recommendation engine suggestions: The app could actively suggest new restaurants based on user ratings and recorded history. In addition, the application could also highlight certain menu items and/or grey out menu items that would not suit the user. If cheese is discovered as a trigger for a migraine from the data analysis, the app could actively steer the user away from restaurants and menu options that contain the trigger. The application could also mine personal datasets to suggest a restaurant that would suit all the members of a group.
5. An unpleasant addition: If all of the above fail to provide sufficient monetary benefit, the application could charge a nominal fee to the users to track incidents of their diseases and access the learning algorithms to mine for hidden patterns underneath their activities to identify the triggers for the disease episode.
6. Expanding the app’s relevance: As a user, I would like to track all of my food consumption and personalize the experience as desired. While the discussion of the article is limited to food at restaurants, it could be easily expanded to other consumables and packaged food products. To facilitate the easy transfer of information from a packaged food to the app, scannable barcodes with relevant nutrient information or image processing of nutrient content could facilitate the experience.
7. Revolutionizing other consumer-facing industries with personalization: Personalization and personalized note taking could revolutionize multiple other industries such as consumer goods (Amazon, local supermarket store), retail (every online retail store you can imagine), technology, vacation and travel spots, financial stock market picks, flights, medical facilities, doctors etc. Every industry that seeks user input to rate their product or service (consumer-facing) could be improved by personalization. These approaches will create heightened user engagement that spell boon for creating products loved by all consumers.
How will this approach help current restaurant recommendation apps?
It is estimated that Yelp receives almost 150 million unique visitors per month to its applications on the desktop and mobile combined. While that is an admirable number, almost 90% of the visitors only view other reviews and do not contribute anything to the platform. The little-known 1/9/90 rule from journalist Susan Kuchinskas’s research suggests that only 1% of users actively write the reviews, 9% of the users edit and/or share them and 90% of the users only visit the site to learn, watch and read without responding [5, 6]. Personalizing the experiences of the 150 million viewers will help Yelp massively increase the number of reviews and improve the 1/9/90 rule.
A recent follow-up study by Eddie Yoon in Harvard Business Review analyzing how many US households like to cook revealed very interesting statistics. The survey indicated that almost 90% of US population dislikes cooking at home and spends thousands of dollars each year eating out and eating precooked meals [7]. Personalization strategies could rapidly capitalize on this trend and involve millions of new users into the food recommendation and propel the user engagement with the app.
These personalization strategies would rapidly improve and expand the data that can be collected by the application and fine-tune its algorithms. These changes would definitely convince me to shell out my dollars and provide my precious data for their analysis algorithms.
Patenting this approach –
While I deeply believe that this change will bring about a positive development in our experiences and hence bring monetary value, I will not be pursuing a patent on this approach or any methods this may lead to. At its heart, patenting allows one to either sell their invention exclusively or prevent a competitor from doing so, neither of which I am considering.
Some claims this approach could lead to include:
- A method to aggregate and mine user-created personalized questions and responses into a generalized database.
- A method of creating an anonymous verified public review from individual personalized questions using language processing.
- A method to change underlying security permissions via toggle between personal and public profiles
Noteworthy changes already happening:
While the listed concerns above indicate the avenues for improvement, there are certain changes I learned during my research that I consider a step in the positive direction. Restaurant recommendation applications now show the public rating of individual menu items. Yelp has started extracting information from individual reviews and then grouping them according to the menu item. One of the benefits of knowing how people have rated individual menu items is to avoid reading through multiple reviews to decide what to order at a restaurant, thereby saving a significant amount of time.
References:
- Finocchi C & Sivori G (2012) Food as a trigger and aggravating factor of migraine. Neurological Sciences 33(1):77-80.
- Fletcher PC & Schneider MA (2006) Is There Any Food I Can Eat?: Living With Inflammatory Bowel Disease and/or Irritable Bowel Syndrome. Clinical nurse specialist 20(5):241-247.
- Millichap JG & Yee MM (2003) The diet factor in a pediatric and adolescent migraine. Pediatric Neurology 28(1):9-15.
- MacDermott RP (2007) Treatment of irritable bowel syndrome in outpatients with inflammatory bowel disease using a food and beverage intolerance, food and beverage avoidance diet. Inflammatory bowel diseases 13(1):91-96.
- https://www.yelpblog.com/2011/06/yelp-and-the-1990-rule
- https://www.americanexpress.com/us/small-business/openforum/articles/the-1990-rule-what-you-need-to-know/
- https://hbr.org/2017/09/the-grocery-industry-confronts-a-new-problem-only-10-of-americans-love-cooking
- Image Copyright: mackoflower / 123RF Stock Photo
9. Image Copyright: kastock / 123RF Stock Photo
Acknowledgements: Author is grateful to Andrew Dobos and Hemanth Kumar Venkateshwara for their help with detailed editing of this article.
Copyright: The work above is the original work of the author and may not be replicated without permission.
Very, very interesting outlook, Sai! If such a Personalization App appears one day, I would hope that the amount of MSG present in the food preparation, as evidenced by one’s later reactions to it, would also be one of the relevant questions included…